Mapping the World with a Global Change Detection AI Model
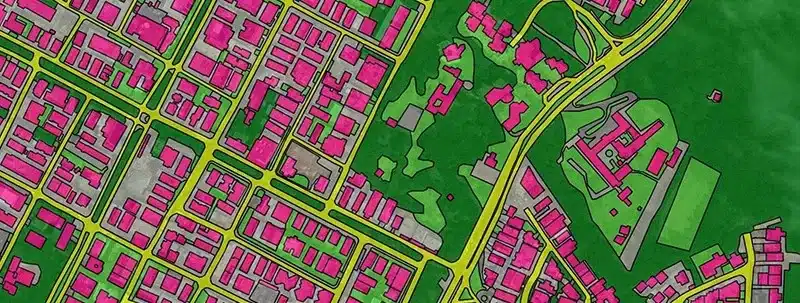
Mapping the world using Global Change Detection AI often involves leveraging artificial intelligence (AI) and satellite or drone imagery to monitor and detect changes in the Earth’s surface over time. This approach allows for rapid, efficient and automated analysis of large-scale geographic areas and provides valuable insights into various environmental, social, and economic phenomena.
Satellite Data Extraction with AI Models
High-resolution satellite imagery is acquired from organizations such as government agencies, commercial providers, or open data repositories. This data may include multispectral or hyper-spectral images captured by satellites constellations e.g. Landsat, Sentinel, or Worldview. The satellites capture images of the Earth’s surface at regular intervals, providing a time series of data. Once acquired, the satellite imagery is preprocessed to correct for atmospheric effects, remove clouds, image alignment, radiometric correction as well as geometric calibration to enhance image quality. The imagery is then georeferenced and aligned to ensure accurate spatial representation.
The Global Change Detection AI algorithms are applied to the preprocessed satellite imagery to identify and analyze changes that have occurred over time. These algorithms often use machine learning techniques, such as convolutional neural networks (CNN), or deep learning models to train a custom model on the labeled dataset and automatically detect patterns and anomalies in the satellite imagery. Training data preparation is then done to create a labeled dataset by manually annotating the satellite images with the objects or features you want to extract. For example, if you’re interested in extracting buildings, you would label the building regions in the images.
The model learns to recognize the patterns and characteristics of the objects you want to extract from the satellite image. During the change detection and analysis, the AI algorithms compare pairs or sequences of satellite images to identify areas where changes have occurred. This can include changes in land cover, vegetation, urban development, water bodies, and other features of interest. The algorithms highlight areas where significant changes have occurred, enabling further analysis and investigation.
After that, you need to assess the performance of the trained model using evaluation metrics including precision, recall, and F1-score. Further, refine the model by adjusting its architecture, hyper-parameters, or increasing the training dataset size, if necessary. The refinement of the extracted objects or features through post-processing techniques like noise removal, filtering, or morphological operations is done. This step aims to improve the overall accuracy and quality of the extracted results.
Data visualization and interpretation is done using the maps or in interactive platforms, allowing users to explore and interpret the results. The maps can display areas of growth, decline, deforestation, urban expansion, or other changes, providing a comprehensive view of the dynamic nature of the Earth’s surface. Lastly, visualize the extracted objects or features on the satellite images or convert them into GIS-compatible formats for further analysis or integration with other geospatial data. The success of image extraction from satellite images using AI heavily depends on the quality and quantity of the training data, the complexity of the objects to be extracted, and the suitability of the chosen machine learning algorithms and techniques.